Making the case for synthetic data

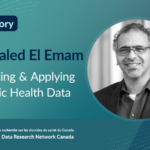
Whether it’s winning fine arts competitions, confusing people with deepfakes or simulating large health data sets, generative AI has been capturing the public’s imagination and causing consternation in equal parts. “Images created using generative AI models have brought into the discourse whether generative AI can be creative. They also raise questions about trust, and how fake data or fake images can be a proxy for real ones,” explained Dr. Khaled El Emam, Canada Research Chair in Medical AI and Professor in the School of Epidemiology and Public Health at the University of Ottawa.
When it comes to health data, he continued, “synthetic data generation is essentially generative AI. You start off with a source data set – it could be a real world data set or a clinical trial data set – and you train a generative model with the aim of learning the simple and complex patterns in the original data. You then use that model to generate synthetic data.”
Synthetic data have become increasingly useful for training machine learning models, software testing and teaching in universities, in part due to the ability to generate large data sets. According to Dr. El Emam, one of the key use cases for synthetic data in health research is privacy. “Because it’s generated from a model, there isn’t a one-to-one mapping between synthetic data and the source data, or between synthetic data and real people, which is why synthetic data can have good privacy properties.” While generative models cannot guarantee that synthetic data will always have low privacy risks, “we can measure the privacy risk of synthetic data because we can quantify those risks reasonably well.”
Images created using generative AI models have brought into the discourse whether generative AI can be creative. They also raise questions about trust, and how fake data or fake images can be a proxy for real ones. ~ Dr. Khaled El Emam
According to Dr. El Emam, the basic principles to train a generative model are the same across different techniques. “You have a generator, like a statistical machine learning model or artificial neural network, that ingests the real data, tries to learn the patterns in the real data and then generates the synthetic data.” An evaluator then compares the synthetic data to the real data and computes utility metrics and privacy metrics, measuring the quality of the synthetic data as well as assessing the privacy risks. “You can compute a score from the evaluator which is fed back to the generator to tune its parameters. You go through this iterative process until you reach a data set that has good performance – either a balance between utility and privacy, or one that maximizes utility only if you’re focusing on that.”
Importantly, you don’t need to know how synthetic data will be used in order to generate useful synthetic data with high utility. “The synthetic data can look very realistic, with the same distribution, variable name, structure and variable types, as well as meet the same conditions as the original data.” Synthetic data can also be useful for bias mitigation, he added. “If you have two groups – say men and women – where the distribution should be equal but you have fewer women in the data set, you take the complete data set and train a generative model. You then simulate additional female patients and add them to the data set. The analysis is performed on the data with simulated patients to augment the underrepresented group in the data set.”
To learn more about Dr. El Emam’s research using synthetic data, watch his presentation as part of HDRN Canada’s Discussion Sessions.